Hey, it's been awhile! We've been unbelievably busy at my company, but I've been plugging away on my genome analysis slowly. When I last blogged, I'd completed the process of identifying small variants in my genome (affecting just one or a few DNA nucleotides). This takes us into an interesting new analysis phase - interpreting the consequences of those variants in the context of existing knowledge of human genetics. I previously went into depth on a certain variant I'd known to look for, but now we'll sift through the others in my VCF file - nearly four million of them!
I began with Ensembl's Variant Effect Predictor (VEP), one of several available tools that annotates VCF variants with their likely consequences for known genes and other genomic features. VEP produces a new VCF file with this additional information crammed into each entry, like so:
1 871215 . C G 1357.81 . AB=0;ABP=0;AC=2;AF=1;AN=2;AO=45;CIGAR=1X;DP=
45;DPB=45;DPRA=0;EPP=4.21667;EPPR=0;GTI=0;LEN=1;MEANALT=1;MQM=60;MQMR=0;NS=1;NUMALT=1;ODDS=63.5608;P
AIRED=1;PAIREDR=0;PAO=0;PQA=0;PQR=0;PRO=0;QA=1543;QR=0;RO=0;RPP=3.44459;RPPR=0;RUN=1;SAF=19;SAP=5.37
479;SAR=26;SRF=0;SRP=0;SRR=0;TYPE=snp;technology.ILLUMINA=1;CSQ=G|ENSG00000187634|ENST00000341065|Transcript|synonymous_variant|140|141|47|P|ccC/ccG|rs28419
423|0.0261008|0.00232558|3/12|||||||1|||SAMD11|HGNC|||G:0.0629|protein_coding|ENSP00000349216|||0.03
|0.08|0.16|0.0026|,G||ENSR00000528855|RegulatoryFeature|regulatory_region_variant||||||rs28419423|0.
0261008|0.00232558|||||||||||||||G:0.0629|||||0.03|0.08|0.16|0.0026| GT:DP:RO:QR:AO:QA:GL
1/1:45:0:0:45:1543:-10,-10,0
That's really not pretty, but VEP also produces a nice series of summary charts. For example, it breaks down putative consequences of my variants in protein-coding sequences.

1 871215 . C G 1357.81 . AB=0;ABP=0;AC=2;AF=1;AN=2;AO=45;CIGAR=1X;DP=
45;DPB=45;DPRA=0;EPP=4.21667;EPPR=0;GTI=0;LEN=1;MEANALT=1;MQM=60;MQMR=0;NS=1;NUMALT=1;ODDS=63.5608;P
AIRED=1;PAIREDR=0;PAO=0;PQA=0;PQR=0;PRO=0;QA=1543;QR=0;RO=0;RPP=3.44459;RPPR=0;RUN=1;SAF=19;SAP=5.37
479;SAR=26;SRF=0;SRP=0;SRR=0;TYPE=snp;technology.ILLUMINA=1;CSQ=G|ENSG00000187634|ENST00000341065|Transcript|synonymous_variant|140|141|47|P|ccC/ccG|rs28419
423|0.0261008|0.00232558|3/12|||||||1|||SAMD11|HGNC|||G:0.0629|protein_coding|ENSP00000349216|||0.03
|0.08|0.16|0.0026|,G||ENSR00000528855|RegulatoryFeature|regulatory_region_variant||||||rs28419423|0.
0261008|0.00232558|||||||||||||||G:0.0629|||||0.03|0.08|0.16|0.0026| GT:DP:RO:QR:AO:QA:GL
1/1:45:0:0:45:1543:-10,-10,0
That's really not pretty, but VEP also produces a nice series of summary charts. For example, it breaks down putative consequences of my variants in protein-coding sequences.
Practical efforts to interpret an individual's genome today focus on variants within such protein-coding sequences, because they're much better understood than RNA genes, introns, regulatory elements, and other non-coding regions. From my 3.7 million genome-wide variants, only a few tens of thousands lie within coding regions, which reflects the overall composition of the human genome. Moreover, more than half of those are synonymous, and therefore rather unlikely to cause important consequences (although I could tell you about some exceptions). That leaves about 30,000 non-synonymous coding variants - still quite a lot to plow through on my own!
To sieve them further, I ran my VEP VCF through GEMINI, which adds additional annotations from existing knowledge databases and other tools, and loads them all nicely into a local SQLite database. This made it easy to query my variants and pull out interesting subsets, like so:
$ gemini query --show-samples --header -q "select * from variants where is_lof=1 or (clinvar_sig is not null and clinvar_sig not in ('non-pathogenic','untested','unknown'))" mlin.db
I thus selected my small variants that are either likely to wreck the encoded protein (loss of function, LOF, usually a stop gain, frameshift, or splice site mutation), or matching an interesting record in ClinVar, a public database of variants of known significance in clinical (medical) settings. This produced a table of 885 variants, and so discarded many thousands of non-synonymous variants that don't clearly have drastic effects on proteins, and aren't known to be clinically significant. Many of those may well be interesting in various ways, but right now they're just very difficult to interpret in isolation.
The 885 remaining are finally a reasonable number to load into that near-universal attractor of bioinformatics data curation, the office spreadsheet.
Notice my ALDH2 variant annotated with "mixed" significance in "acute alcohol sensitivity" and "alcohol dependence" - makes sense. But since I don't seem to suffer from 884 other notable genetic conditions, what to make of the rest? Well, there's quite a lot more to consider at this stage:
Stepping back: why sequence someone's whole genome - billions of DNA nucleotides - and then look at only a few dozen carefully? Well, this approach makes sense if we aren't looking for any specific known genetic condition or risk factor. So we collect everything, and then look at what seems most interesting with what knowledge and resources we have. All those millions of variants I skipped over ruthlessly, because we're not good at making sense of them yet, will still be there in the future! The field is advancing so quickly that there will be much more to learn if I revisit them in a few years. Furthermore, DNA sequencing technology advancements will hopefully give me a better look at structural variants in addition to the small variants we're now pretty good at detecting.
Next time, I'll write a few interesting vignettes from the individual curation of my small variants.
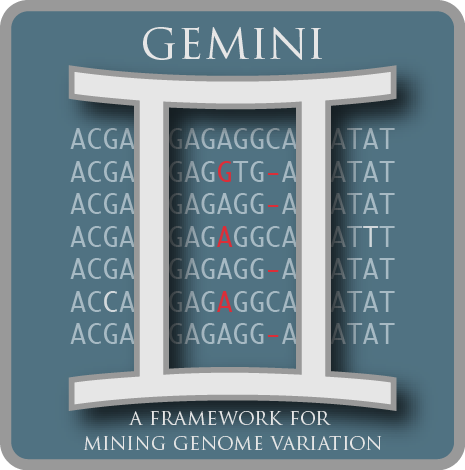
$ gemini query --show-samples --header -q "select * from variants where is_lof=1 or (clinvar_sig is not null and clinvar_sig not in ('non-pathogenic','untested','unknown'))" mlin.db
I thus selected my small variants that are either likely to wreck the encoded protein (loss of function, LOF, usually a stop gain, frameshift, or splice site mutation), or matching an interesting record in ClinVar, a public database of variants of known significance in clinical (medical) settings. This produced a table of 885 variants, and so discarded many thousands of non-synonymous variants that don't clearly have drastic effects on proteins, and aren't known to be clinically significant. Many of those may well be interesting in various ways, but right now they're just very difficult to interpret in isolation.
The 885 remaining are finally a reasonable number to load into that near-universal attractor of bioinformatics data curation, the office spreadsheet.
Notice my ALDH2 variant annotated with "mixed" significance in "acute alcohol sensitivity" and "alcohol dependence" - makes sense. But since I don't seem to suffer from 884 other notable genetic conditions, what to make of the rest? Well, there's quite a lot more to consider at this stage:
- I have two copies of nearly all genes, from my mother and father. If only one copy of a given gene is busted, there might be reduced or no consequence.
- Even two broken copies of a given gene isn't necessarily that bad; many genes exist in gene families, or participate in elaborate regulatory networks, which probably have some degree of robustness. Some genes have rather trivial functions or do nothing really useful at all.
- Many of the LOF variants are widespread among people of my ethnicity. That makes it unlikely they're really terrible, else they'd have been selected out - although, one could dispute this in adult-onset diseases.
- The evidence and reports underlying ClinVar records are sometimes mixed or conflicting, and frequently revised.
- The annotations of gene structures, which underlie the LOF annotations, are similarly never quite complete.
- Some errors in the sequencing and variant calling pipeline will have slipped through.
Stepping back: why sequence someone's whole genome - billions of DNA nucleotides - and then look at only a few dozen carefully? Well, this approach makes sense if we aren't looking for any specific known genetic condition or risk factor. So we collect everything, and then look at what seems most interesting with what knowledge and resources we have. All those millions of variants I skipped over ruthlessly, because we're not good at making sense of them yet, will still be there in the future! The field is advancing so quickly that there will be much more to learn if I revisit them in a few years. Furthermore, DNA sequencing technology advancements will hopefully give me a better look at structural variants in addition to the small variants we're now pretty good at detecting.
Next time, I'll write a few interesting vignettes from the individual curation of my small variants.
Major industries in the country are engineering, electronics, wood and wood products, textiles, information technology, telecommunications. The total labor force of Estonia is 670,200 people, wherein 5.5% of population in the country are unemployed. The total number of unemployed people in Estonia is 71,873. The Industrial Production growth rate of Estonia is 10%.
ReplyDeleteDo you want to buy papers online and take a break from your student routine? If so, then an excellent online service that has earned the trust of students will help you with this.
ReplyDeleteDo my college homework for me offers you the most affordable but excellent custom writing services & assistance by professional qualified writers of USA. do my college homework for me
ReplyDeleteOur experts take every minute detail about the Essays into concern and offer with superiority paper. Nursing Essay Writing Service UK
ReplyDeleteBut that’s not asset protection
ReplyDeleteenough when we start talking about the importance of hiring residential security services in London. These have been summarised here in this blog.
your material sounds promising! your research is very important. keep up the good work. and if you need writing help, then contact the company's specialists pay for essay who are the best in the niche for writing articles and dissertations to order
ReplyDeleteIf you have a question regarding your order or the help itself, you can read her latest blog or contact our 24/7 customer support via phone, email or online chat. You can discuss the order and easily track its progress right through the website 24/7.
ReplyDeleteI see you have done a great job, it is worthy of praise. I think that you could do more if you had better information. it can be gleaned from the articles of the authors of the company paperswriting.services who have written tons of material on various topics and have clearly affected your
ReplyDeleteThis comment has been removed by the author.
ReplyDeleteWith cute and lovable balloons, sweet candy and gifts that will show and interest them if they get the https://kids-math-games.com/number-games/ right answers! Just drag and drop the correct answer to each equation and solve the puzzle! This is a specially built educational game for toddlers that will keep their focus on learning math.
ReplyDeleteWe are assignment in need, a guaranteed organization to give you the best offices to your pay someone to write a research paper task at a reasonable cost. For our purposes, reasonable doesn't mean compromising with quality.
ReplyDeleteGood morning! Writers of team from https://us.grademiners.com/ can write your essay cheap and fast. Deadlines vary from 1 hour to 30 days, sometimes more, which mostly depends on the type of paper. An essay can be done within hours, while a dissertation could require up to 2 months to do well from scratch.
ReplyDeleteTo date, the multiplayer online battle arena (MOBA) has become a widely popular gaming genre. This multiplayer game genre often needs five players to join a team and compete against another five-player team. The most popular MOBA games are known for being a competitive genre in which players must work together to succeed. In reality, this genre necessitates abilities and technique to challenge individuals all around the world. While MOBAs are popular on mobile devices these days, many people prefer the larger screen that PCs provide. When playing on smartphones, issues such as accidently hitting another button when you meant to click another occur.
ReplyDeleteLearn How to Play Castle Clash on PC | Games.lol
Go to Slope Wallet official website and select from Android or iOS for mobile application and select Chrome for desktop. You can also go directly to the Chrome Store, Google Play, or App Store. Then, search "Slope Wallet" and install.
ReplyDeleteAtomic Wallet |
인천콜걸
ReplyDelete광주콜걸
인천콜걸
충남콜걸
충남콜걸
세종콜걸
세종콜걸
서울콜걸
ReplyDelete울산콜걸
대구콜걸
대전콜걸
인천콜걸
세종콜걸
부산콜걸
인천콜걸
울산콜걸
대구콜걸
"Social media platforms provide valuable analytics and insights to help bloggers track their growth." kristel staci
ReplyDeletePROJ6000 Principles of Project Management
ReplyDeleteGet PROJ6000 Principles of Project Management Assignment Help with Punjab Assignment Help at an affordable price and timely delivery.
MBA Assignment Help
ReplyDeleteGet MBA Assignment Help at Latrobe University with Punjab Assignment Help at an affordable price and timely delivery. We have Experts on the team.
MBA Assignment Help
ReplyDeleteNice Article and I appreciate your thought and views. This is really great work. Thank you for sharing such a useful information here on the blog. If you need any support for QuickBooks desktop error 6189 816 then get immediate help from our QuickBooks Experts.
This comment has been removed by the author.
ReplyDeleteTime is of the essence in medical emergencies, and Air Ambulance Services in Raipur understand the urgency. They offer rapid response times, ensuring that patients receive timely medical attention and transport to appropriate healthcare facilities. Air Ambulance Services In Raipur
ReplyDeleteIn times of medical emergencies, every second counts. Access to prompt and efficient medical care can make a significant difference in saving lives. This is where Air Ambulance Services in Patna play a crucial role in providing swift and specialized medical assistance to those in need. Air Ambulance Services In patna
ReplyDeleteAir Ambulance services in Jabalpur are available 24/7, providing quick response times and rapid transportation to medical facilities. The services can be booked in advance or arranged on short notice, depending on the urgency of the situation. They offer a safe and comfortable environment for patients during transportation, ensuring they receive the best possible medical care and attention. Air Ambulances Service in Jabalpur
ReplyDeleteThis comment has been removed by the author.
ReplyDeleteAir ambulance services in Bihar provide rapid and specialized medical transport for critically ill or injured patients. Equipped with advanced medical equipment and staffed by trained professionals, these services ensure timely and safe transfer to hospitals or specialized treatment centers. They are essential for overcoming geographical barriers and providing immediate medical attention, significantly improving the chances of survival and recovery in emergency situations. Air ambulance services in Bihar
ReplyDeleteComplete Laundry Care” provides comprehensive car cleaning services in Noida, focussing on maintaining the cleanliness and appearance of vehicles. Bag Cleaning Services | Car Cleaning Services | Carpet Cleaning Services | Dry Cleaning Services | Organic Dry Cleaning | Shoe Cleaning Services | Sofa Cleaning Services | Steam Press Services
ReplyDeleteReady to dive into the world of trading? Just pop over to the Robinhood login page, slap in that email or username, and watch the doors to your portfolio swing open! Password? Check! Got two-factor authentication? Even better – your investments are safe and sound! With just a few clicks, you’re in the driver’s seat, ready to surf the stock and crypto waves.
ReplyDeleteLog In | Robinhood® - Sign In to Your Account
Navigate Lufthansa Flight Cancellation Policy effortlessly with Tours N Travel Pro. Learn about flexible cancellations, refund eligibility, and rebooking options. Whether you managing a last-minute change or seeking a refund, our guide ensures a seamless experience with Lufthansa. Plan stress-free travel with expert insights from Tours N Travel Pro.
ReplyDeleteAt Tours N Travel Pro, we simplify the Alaska Airlines Cancellation Policy for you. Discover flexible cancellation terms, 24-hour refunds, and credit usage guidelines. Whether plans change last minute or you managing unforeseen circumstances, our guidance ensures smooth rescheduling. Trust us for hassle-free travel solutions.
ReplyDeleteThe Damac Cavalli Estates is a modern home comprising 6 to 7 BHK bedroom villas filled with high-end amenities. You can think of any modern luxury facility that will be at your doorstep.Since this place is located in the Dubai Hills, providing you with exclusive conductivity facilities to maintain your exquisite lifestyle.
ReplyDeleteDamac Cavalli Estates
Ledger.com/start a global leader in crypto security, utilizes advanced blockchain technology to protect your assets from online threats. With Ledger hardware wallets, your private keys remain offline, reducing the risk of hacks and unauthorized access.Getting started is simple. Visit Ledger.com/Start to set up your Ledger Nano X or Ledger Nano S Plus. The step-by-step guide will walk you through the initialization process, Ledger Live installation, and secure recovery phrase setup
ReplyDeleteCandolim Beach
ReplyDeleteCalangute Beach
Baga Beach
ReplyDeleteLatam America in Center
Tron ride Lightcycle Run
ReplyDeletehow to delete facebook account from app
deepseek coder v2
ReplyDeletebest crypto to buy now
Robinhood layoffs
ReplyDeleteGemini AI Login
Embarking on your cryptocurrency journey with Trezor begins at trezor.io/start, the official portal designed to guide you through the setup and secure management of your digital assets.
ReplyDeleteVisit trezor.io/start | to securely set up your Trezor hardware wallet. Follow an easy step-by-step guide to install Trezor Suite, back up your recovery seed, and protect your crypto assets from online threats. Start your journey to secure cryptocurrency storage today.
ReplyDelete